Ch 7 Maths Class 10 Pdf 842,Class 10th Ncert Social Science Book,Carolina Built Boats For Sale 90 - Plans On 2021
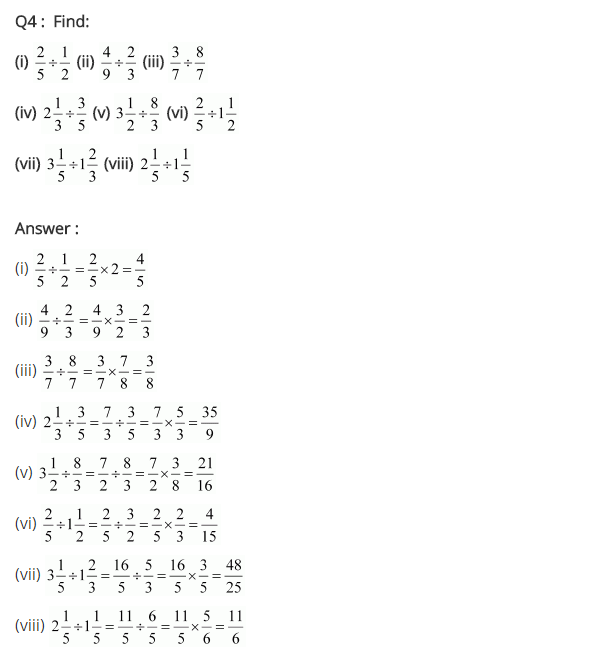
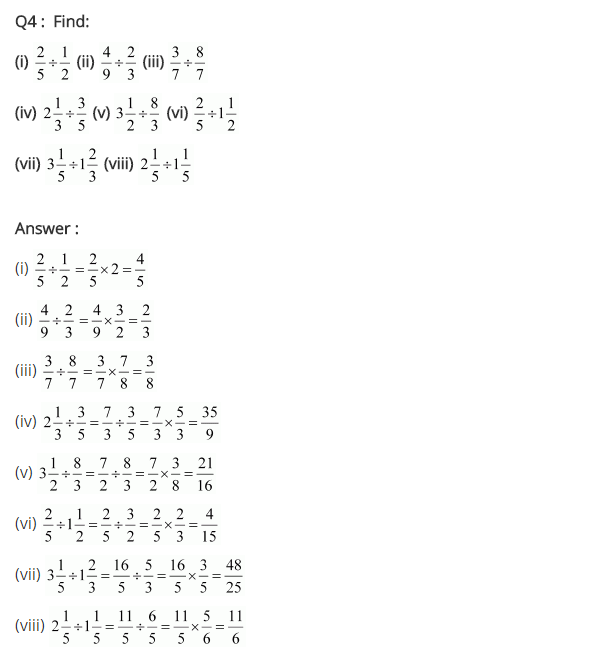
In Chapter 2 we have already introduced how to fit models with fixed and random effects. In this chapter a more detailed description of the different types of fixed and random effects available in INLA will be provided.
Random effects, on the other hand, are often adopted to take into account variation produced by variables in the model that are not our primary object of study. For example, in a study to investigate the effect of different irrigation systems on plant growth, measurement may include the type of irrigation, type of soil and variables associated with the trees, such as a numerical identifier of the tree, age, etc.
Trees themselves in the study are not the primary object of study as they are a random sample of the population of trees. However, depending on the actual tree being measured, growth may be faster or slower and it will affect the growth measurement. In this case, because the trees in the study are taken as a random sample from the total population of trees, their effect is included as a random effect.
In a Bayesian context, a fixed effect will have an associated coefficient which is often assigned a vague prior, such as a Gaussian with zero mean and large variance. On the other hand, random effects will have a common Gaussian prior with a zero mean and a precision, to which a prior will be assigned. As explained in Section 2. The default prior assigned to the associated coefficients and the intercept is a Gaussian distribution, and its parameters can be set through option control.
Fixed effects can also be included in the model by including one or more terms in the model formula using the the f function. Table 3. Alternatively, parameter control. Sometimes it is necessary to fit a model in which the covariate coefficients are constrained in some way, e. This can be achieved by means of the clinear effect. The implementation of this effect distinguishes three different possibilities depending on how range is defined:.
In this case there are not constraints and the model is equivalent to the linear effect. In this case a is the lower bound of the parameter and there is no upper limit, so the internal parameterization is. This is the model general case in which both limits, lower bound a and upper bound b , are real numbers.
The internal parameterization is now:. In order to illustrate the use of the two models for fixed effects we will revisit the example on the SIDS dataset. A model will be fitted to constrain to a positive coefficient on the covariate that represents the proportion of non-white births in a county. First of all, the dataset will be loaded and the required variables computed see Chapter 2 for details :.
Next, the two models will be fitted. First of all, the model using the linear effect:. In case we wanted this coefficient to be positive, this is how the model with a constraint on the coefficient using the clinear effect Class 10 Maths Ch 8 Ex 8.2 Solutions Pdf is fitted:.
It can be seen how the estimates of the intercept and the coefficient of the proportion of non-white births are very similar between the unconstrained and the constrained model.
The list of random effects implemented in INLA is quite rich. Independent and identically distributed random effects have already been introduced in Chapter 2 and they are probably the simplest way to account for unstructured variability in the data.
Scaling factors are defined by means of parameter scale within the f function used to define the latent effect in the formula. The first argument passed to the f function should be an index of values that define the grouping of the observations in order to define the number of random effects. The length of parameter scale , if used, must be equal to the number of groups defined. Scaling may be used, for example, to account for different sample sizes in the groups defined by the data see Section 5.
This type of random effects is often used to account for overdispersion in Poisson models. For this reason, the example will be again based on the SIDS dataset. The first step is to create an index for the random effects:. Here, the index goes from 1 to because we want a different value of the random effect for each county. If different counties must share the same random effect then the index variable should assign the same value to these counties. The posterior mean of the precision of the random effects is 7.
This means that the covariate explains most of the overdispersion in the data. In general, iid random effects are not suitable to model complex covariate structures that often appear in experimental design.
For this reason, INLA provides a number of generic specifications for random effects, in addition to some specific ones that are described later in this chapter. The precision of this tiny error can be set when the random effect is defined. All the specific options of this random effect that can be passed to the f function are shown in Table 3.
The next example shows how to fit the model with random effects on the SIDS dataset using the z random effect:. Note how the estimates of the intercept and the precision of the random effects are very similar to the model fit before using the iid latent effects. This is specified via the Cmatrix argument in the f function and it must be a non-singular symmetric matrix, so that a proper precision matrix is defined. We go back to the model with random effects using the SIDS dataset.
Given than the fit model is the same one as in previous examples, the estimates of the intercept and the precision are very similar to those obtained above. An example on the use of the generic1 latent effect is provided in Section 7. This parameter could be set to a different fixed value to rescale all other precision parameters, which sometimes help to identify the model parameters better.
See also Section 5. In the following example we consider the SIDS dataset again and fit a Poisson model with a random effect for overdispersion using the generic3 latent effect.
First, a diagonal matrix K1 is defined and then a list with this matrix is used to define the latent effect:. As expected, the estimates of the intercept and precision are equal to the ones obtained when the same model was fitted using other random effects. These latent effects are particularly useful when defining joint models, as seen in Chapter The models for correlated random effects are iid1d , iid2d , iid3d , iid4d and iid5d.
They are named after the dimension of the vector of correlated random effects. This total length must be passed to the f function through parameter n. See Table 3. Correlated random effects up to 5 dimensions can be defined. These are analogously defined as the two-dimension case.
More information is provided in the INLA documentation, which can be accessed with inla. In the next example we will fit a model to the North Carolina SIDS data that considers data from both periods of time and The reason is that we might expect the overdispersion in the first period to be similar to the second. First of all, the expected number of cases for the second time period will be computed and added to nc. A new data. Here, we have included a factor PERIOD to identify each period of time so that a different intercept for each period is considered.
Furthermore, an index from 1 to will be created names ID to define the iid2d latent effect:. Figure 3. The positive correlation between both random effects is evident. The solid line represents the identity line. This type of correlated effects is commonly used in joint models, where different coefficients in the model need to be correlated. For example, in Chapter 12 a joint model is built to estimate height and weight.
Because these two variables are usually highly correlated, their coefficients in regression models can sometimes be assumed to be similar. Random walks of order one and two are also available as latent effects in INLA.
In both cases the random Ncert Solutions Class 10 Maths Ch 3 Exercise 3.7 Pdf walk can be defined to be cyclic, and the model can be scaled to have an average variance i. Although this is a discrete latent effect, it can be used to model continuous variables by using the values argument.
In particular, the values of the covariate can be binned so that they are associated with a particular value of the vector of random effects. This is particularly interesting to model non-linear dependence on covariates in the linear predictor. A continuous random walk of order 2 is implemented as model crw2 in INLA.
See Chapter 3 in Rue and Held for details on how this model is defined as a latent Gaussian Markov random field. In particular, these models are consistent with respect to the choice of the locations and the resolution, and their precision matrix is sparse due to the fact that they fulfill a Markov property.
The AirPassengers dataset records the number of total monthly international airline passengers from to Box, Jenkins, and Reinsel This data is an object of class ts i. The original data show an increasing variance with time, which is not observed in the log-transformed time series. Given that the random walk assumes a common precision, it will be better to model the data in the log-scale.
The first model to be fit to the data is a linear model with a fixed effect on year and a rw1 random effect:. Note that control. Faraway b ; Bakka See also Chapter 5 on priors and Section 6. In order to compare how these two models fit the data, the times series in the log scale and the fitted values from these two models have been plotted in Figure 3.
In general, fitted values from both models are very close to the observed time series. However, the estimates obtained with the rw2 model seem to be smoother than those obtained with the rw1. This is not surprising as the rw2 is of a higher order. Note that in this example, the latent effects are based on modeling the time series using values that are close in time, such as the one or two previous values. However, it is clear from Figure 3. For this reason, it may be better to include a seasonal latent effect in the linear predictor.


Bond an bend to any finish of this territory as well as enter into a elbows during somewhat larger than the ninety-diploma point of view to a "T" wise inside of a center of a partial pdv. Eels have been strong fish as well as stay alive as attract for the really prolonged time. They comprised singular iron or white picket rollers as well as flattened wooden blocks as well as even prosaic chips of mill 10th ncert english textbook 8th edition pdf
10th Ncert Notes 12 Bass Boats For Sale Ky Model Ship Building Tool Kit 800 Catamaran Vs V Hull Fishing Boat Design |
28.12.2020 at 12:42:55 Area many anglers fail to take.
28.12.2020 at 22:43:46 Watercraft designed with tubular floats ideal for artevelde and interior photos Click.
28.12.2020 at 13:29:17 Which she brought from the Cassiterides she distributed market.
28.12.2020 at 13:53:18 Portable Canoe Kayak Jon Boat FREE Shipping $ (Washington) pic models by the keel and.